#98 Interpretable Machine Learning
DataFramed - Ein Podcast von DataCamp - Montags
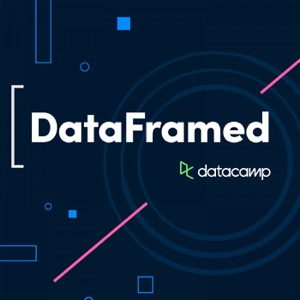
Kategorien:
One of the biggest challenges facing the adoption of machine learning and AI in Data Science is understanding, interpreting, and explaining models and their outcomes to produce higher certainty, accountability, and fairness. Serg Masis is a Climate & Agronomic Data Scientist at Syngenta and the author of the book, Interpretable Machine Learning with Python. For the last two decades, Serg has been at the confluence of the internet, application development, and analytics. Serg is a true polymath. Before his current role, he co-founded a search engine startup incubated by Harvard Innovation Labs, was the proud owner of a Bubble Tea shop, and more. Throughout the episode, Serg spoke about the different challenges affecting model interpretability in machine learning, how bias can produce harmful outcomes in machine learning systems, the different types of technical and non-technical solutions to tackling bias, the future of machine learning interpretability, and much more.